Projects and Research Topics
Roseau cane die-back in Louisiana coasts (2020~present)
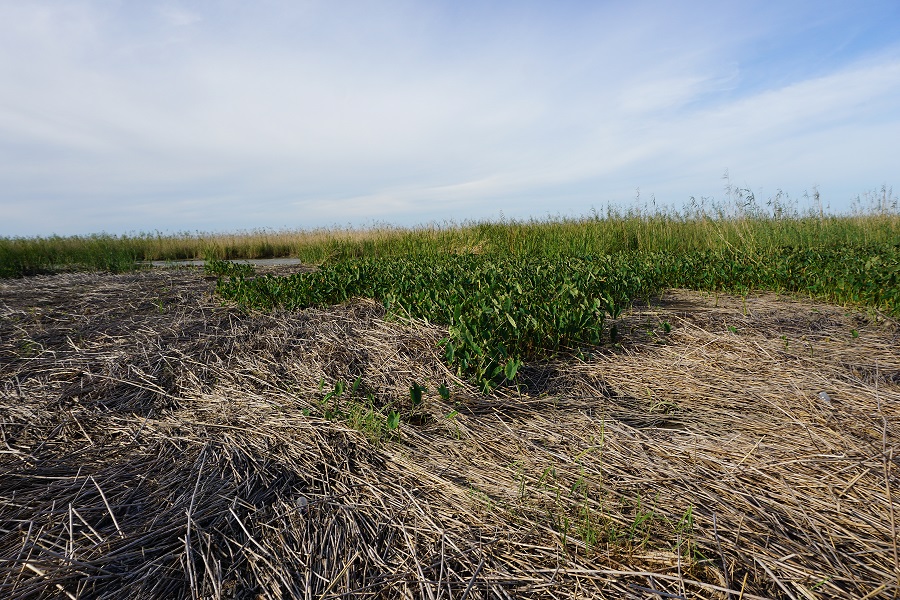
Roseau cane die-off in the Mississippi River Delta in the fall of 2021.Photo Credit: Xuelian (Shelley) Meng
In a state with well-known rapid land loss, Roseau cane plays a significant role in stabilizing Louisiana coasts because of its salt- and inundation-tolerant characteristics, deep root systems, and wide coverage. However, severe Roseau cane die-back have gained increasing attention following the first official reporting of scale infection in the region in 2016. With Roseau cane as the sole feeding resource, scales colonize on their stems and propagate to larger clusters, which is one of the major factors that has caused Roseau cane die-back and die-off in the Mississippi River Delta (MRD) and may have affected the region earlier than 2016.
The Animal and Plant Health Inspection Service (APHIS) of the United States Department of Agriculture (USDA) funded a group of research teams from LSU and other organizations to conduct a comprehensive investigation and assessment on Roseau cane die-back. Our team joined the project from the fall of 2020 and focused on utilizing remote sensing techniques to assess the Roseau cane die-back from different scales. Specifically, our team members assess wetland die-back back and Roseau cane die-back in the lower MRD region through two decades of Landsat images. In addition, we conducted high-resolution Roseau cane habitat mapping covering two of the MRD main outlets. Through flexible drone operations with 10-band cameras, we conducted fine scale habitat mapping and monitoring through a drone site in Pass-A-Loutre outlet in MRD and captured the impact from Hurricane Ida and wetland restoration.
UAV-based vegetation habitat and microtopography mapping in densely vegetated environment for wetland restoration
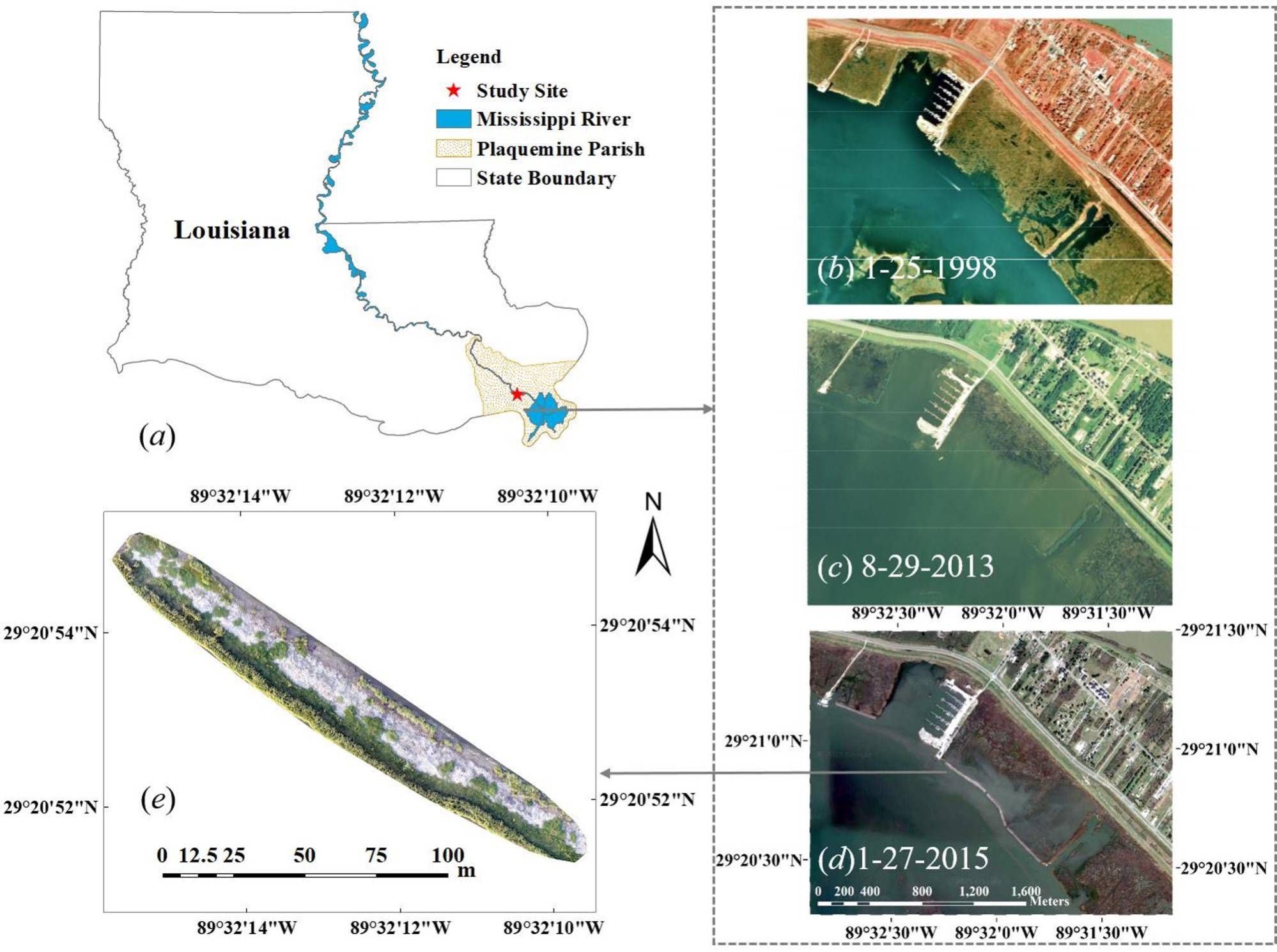
Wetland restoration site.Photo Credit: Meng et. al. 2017
Photogrammetric UAV sees a surge in use for high-resolution mapping, but its use to map terrain under dense vegetation cover remains challenging due to a lack of exposed ground surfaces. This paper presents a novel object-oriented classification ensemble algorithm to leverage height, texture and contextual information of UAV data to improve landscape classification and terrain estimation. Its implementation incorporates multiple heuristics, such as multi-input machine learning-based classification, object-oriented ensemble, and integration of UAV and GPS surveys for terrain correction. Experiments based on a densely vegetated wetland restoration site showed classification improvement from 83.98% to 96.12% in overall accuracy and from 0.7806 to 0.947 in kappa value. Use of standard and existing UAV terrain mapping algorithms and software produced reliable digital terrain model only over exposed bare grounds (mean error = -0.019 m and RMSE = 0.035 m) but severely overestimated the terrain by ~80% of mean vegetation height in vegetated areas. The terrain correction method successfully reduced the mean error from 0.302 m to -0.002 m (RMSE from 0.342 m to 0.177 m) in low vegetation and from 1.305 m to 0.057 m (RMSE from 1.399 m to 0.550 m) in tall vegetation. Overall, this research validated a feasible solution to integrate UAV and RTK GPS for terrain mapping in densely vegetated environments.
Reference:
Meng, X., N. Shang, X. Zhang, C. Li, K. Zhang, X. Qiu, and E. Weeks. 2017. Photogrammetric UAV mapping of terrain under dense vegetation: An object-oriented classification ensemble algorithm for classification and terrain correction. Remote Sensing, 9(11): 1187, DOI: 10.3990/rs9111187.
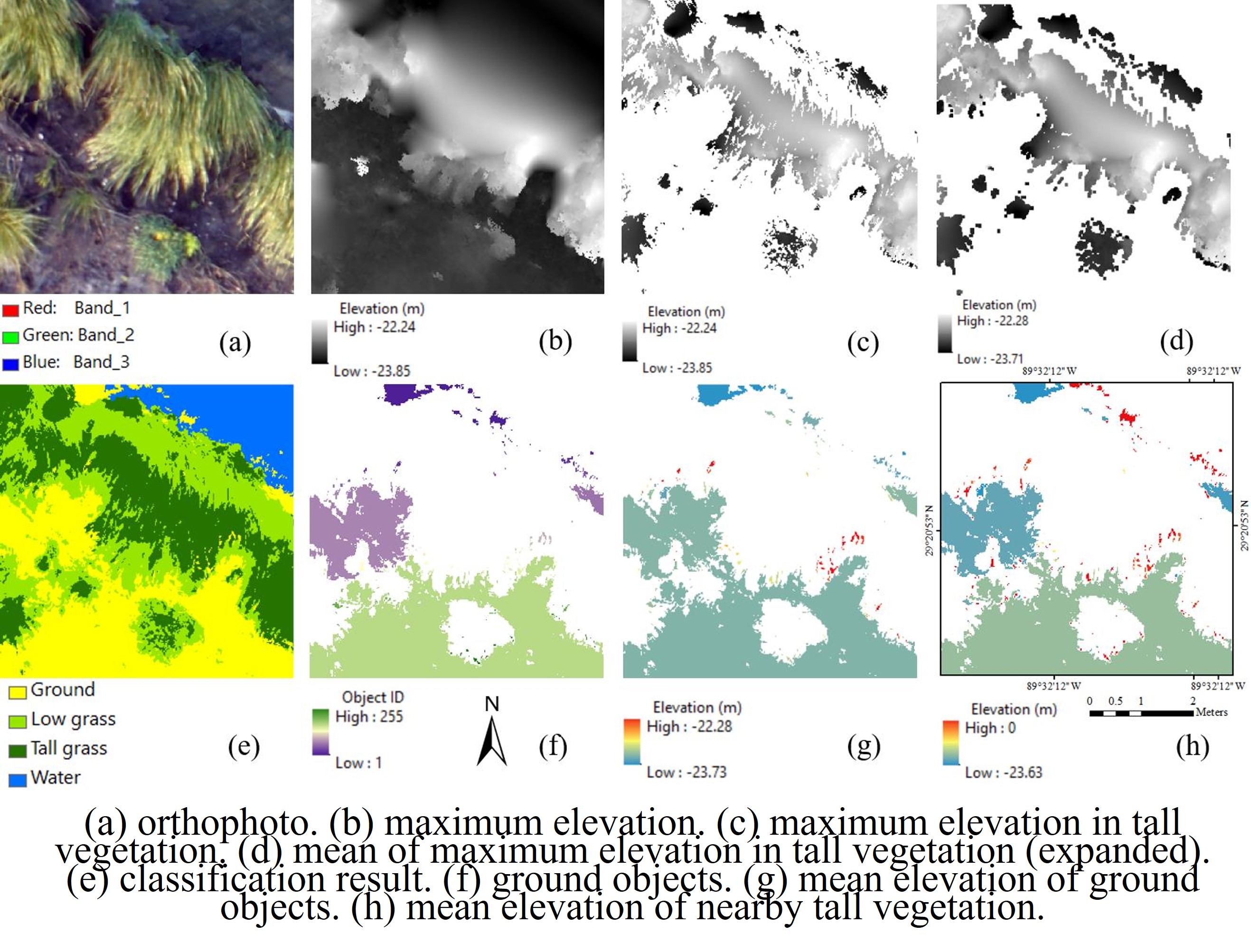
Object-oriented parameters from natural color UAV images.
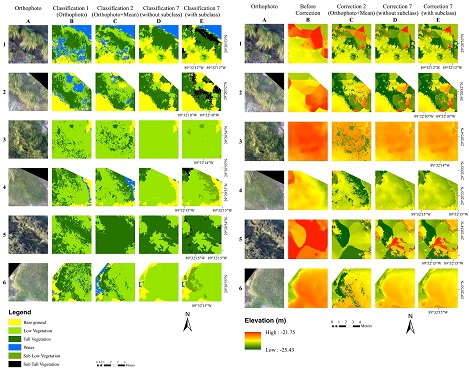
UAV-based vegetation classification correction and terrain correction.
Note: more projects under construction